Young Mok Jung | PhD
tom418@alumni.kaist.ac.kr | Google Scholar | Linkedin | CV
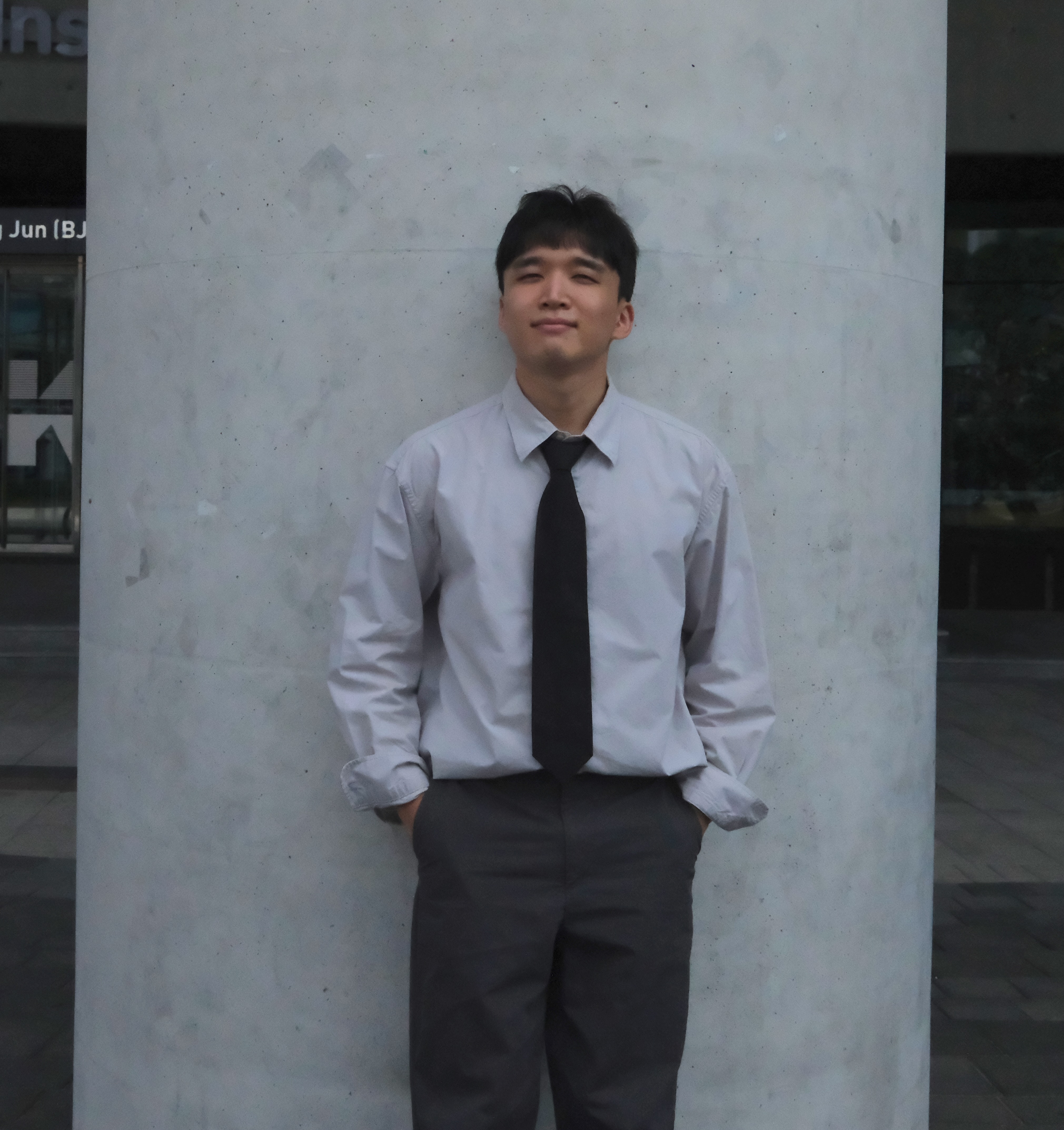
I am an AI & Data team lead at Institute of Genome Insight (Inocras), where I collaborate with geneticists to develop secondary and tertiary analysis solutions for genomics data, aiming to uncover novel biological insights to enhance human health.
I received my PhD in Electrical Engineering from KAIST, where I was advised by Dongsu Han and Young Seok Ju. My dissertation introduced new methods for improving genome analysis with AI and ML. Before then, I received my BS degree in Electrical Engineering from KAIST, South Korea.
My interests are in crafting high-performance systems that incorporate AI/ML approaches. I concentrate on utilizing underlying assumptions of real-world systems to enhance the integration of AI/ML techniques. My work aims at:
- Designing efficient systems built on AI/ML approaches.
- Improving AI/ML approaches by exploiting system-specific assumptions.
All my work involves several months of implementation followed by thorough testing in real-world data.
Recently, I am striving to develop secondary and tertiary analysis solutions for genomics data using AI/ML approaches. Concurrently, I have worked on various topics in high-performance networked systems, including AI-enhanced video delivery systems and data-center networking.
Keywords: Deep-learning and Machine-learning, Genomics software, High-performance network systems
selected publications
- SIGCOMMNeural-Enhanced Live Streaming: Improving Live Video Ingest via Online LearningIn Proceedings of the Annual Conference of the ACM Special Interest Group on Data Communication on the Applications, Technologies, Architectures, and Protocols for Computer Communication, Virtual Event, USA, 2020
Resume
Work
-
2023.08 - Present AI Tech lead
Inocras .Inc (formally Genome Insight .Inc)
Leveraging AI and ML to transform genome analysis into a scalable, data-driven approach capable of handling million-genome scale data
- AI/ML
- Generative AI
- Genomics
- Deep learning
- Drug discovery
- Cancer
- High-performance computing
Education
-
2018.09 - 2024.02 Daejeon, South Korea
Ph.D.
Korea Advanced Institute of Science and Technology (KAIST)
Electrical Engineering
- Distributed network system
- High-performance computing
- Graph signal processing and Graph deep learning
- Deep Reinforcement Learning
- Deep learning for Image Restoration and Quality Enhancement
- Data Mining
- Big data
-
2016.06 - 2016.09 Denmark
-
2014.03 - 2018.08 Daejeon, South Korea
Awards
- 2023
Samsung Humantech Paper Award
Samsung Electronics
Awarded Silver-prize (118 out of 1972 papers) for the paper titled 'Co-optimizing for Flow Completion Time in Radio Access Network' at the 2023 Samsung Humantech Paper Award.
- 2022
Samsung PhD Sponsorship
Samsung Electronics
Awarded full scholarship for Ph.D. program at KAIST by Samsung Electronics.
- 2020
1st Place in Kiwoom US Stock Trading Competition
Kiwoom
Secured 1st place out of 10,000 participants in Kiwoom’s US Stock Trading Competition, achieving a 201% return on investment over 11 weeks. Consistently maintained a similar ROI (180-200%) in the subsequent two competitions, placing in the top 50.
Skills
AI & ML | |
Deep learning for Image data | |
Semi-supervised learning | |
Domain adaptation | |
Graph neural network | |
reinforcement learning | |
Machine learning |
Computer System | |
Distributed system | |
High-performance computing | |
Networked system | |
Memory-efficient system | |
Cloud | |
Data-center networking |
Genomics | |
Read alignment algorithm | |
Indel realignment | |
Small Variant Calling | |
Copy-number alteration | |
Structural variant calling |
Languages | |
English - Fluent | |
Korean - Native |
Projects
- 2018.09 - 2020.03
Live-NAS: Stream high-quality live videos even when the network becomes congested
- Live video accounts for a significant volume of today’s Internet video. Despite a large number of efforts to enhance user quality of experience (QoE) both at the ingest and distribution side of live video, the fundamental limitations are that streamer’s upstream bandwidth and computational capacity limit the QoE of thousands of viewers.
- We developed neural-enhanced live video streaming system (LiveNAS) that incorporates 1) Online training and inference system for super-resolution DNN model during live video streaming. 2) Bandwidth allocation algorithm to maximize user QoE
- LiveNAS delivers live video with the same quality as Google WebRTC using only 45.9% bandwidth on average. LiveNAS system is built on top of Google WebRTC C++ code.
- 2020.03 - 2022.06
BWA-MEME: Machine-learning Enhanced Read Alignment Software
- BWA MEM is a industry-standard alignment software for next-generation sequencing data developed by the Broad Institute of MIT and Harvard.
- We developed BWA-MEME that achieves up to 3.45x speedup in seeding throughput over its' predecessor, BWA-MEM2 from Intel and Broad institute, while ensuring identical output.
- BWA-MEME is now operational in the production environments of numerous institutions, projected to lower alignment costs by 35%. This efficiency translates into millions of $ in cost reductions for projects on a million-genome scale.
- 2022.07 - 2023.08
Generalizing Deep Variant Callers via Domain Adaptation and Semi-supervised learning
- Deploying deep learning-based variant callers (DVCs) to a sequencing method with varying error profiles necessitates generalization which is challenging due to their reliance on extensive labeled data.
- We developed a generalization framework that enables deep learning-based variant callers (e.g., Google DeepVariant, Clair3) to accommodate diverse sequencing methods, leveraging semi-supervised learning and domain adaptation techniques.
- RUN-DVC improves SNP F1-score and INDEL F1-score by up to 6.40 %p and 9.36 %p over the supevised training approach using only unlabeled data or achieves the same variant calling accuracy using merely half of the labeled data.